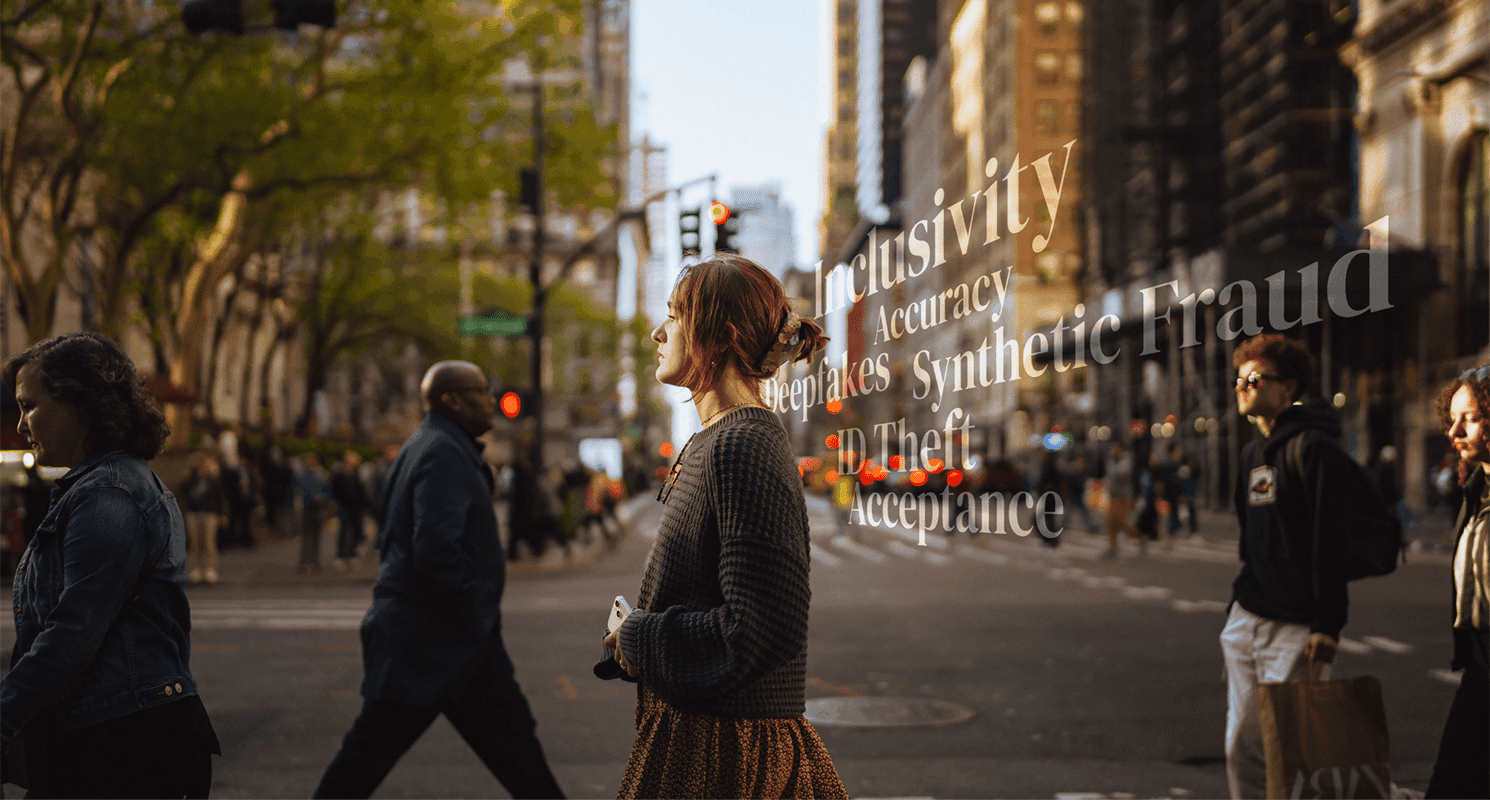
Certainty in identity
transforms onboarding
Socure’s AI powers risk and trust decisions that let you say ‘yes’ to more trusted consumers at every touch point.
Identity, fraud, AML, and trust solved with a single platform
Achieve identity certainty with a best-in-class stack that powers one to many solutions tailored to your risk profile for real-time decisions that let you combat fraud and say ‘yes’ to more new and returning users.
The Path to Identity Certainty with SocureID
With every digital interaction, Socure’s dynamic 360-degree view of identity only gets more accurate, powering every real-time risk and trust decision.
Stop identity compromise at every point along the customer journey
From onboarding to login and account management, the right identity trust decisions can stop fraud and help ensure a seamless customer experience.
Onboarding
Making the right decisions for a consumer you’ve never seen before shouldn’t be complicated.
Contact center
Bring trust to the contact center, so you can focus on good CX.
Discover your solution.
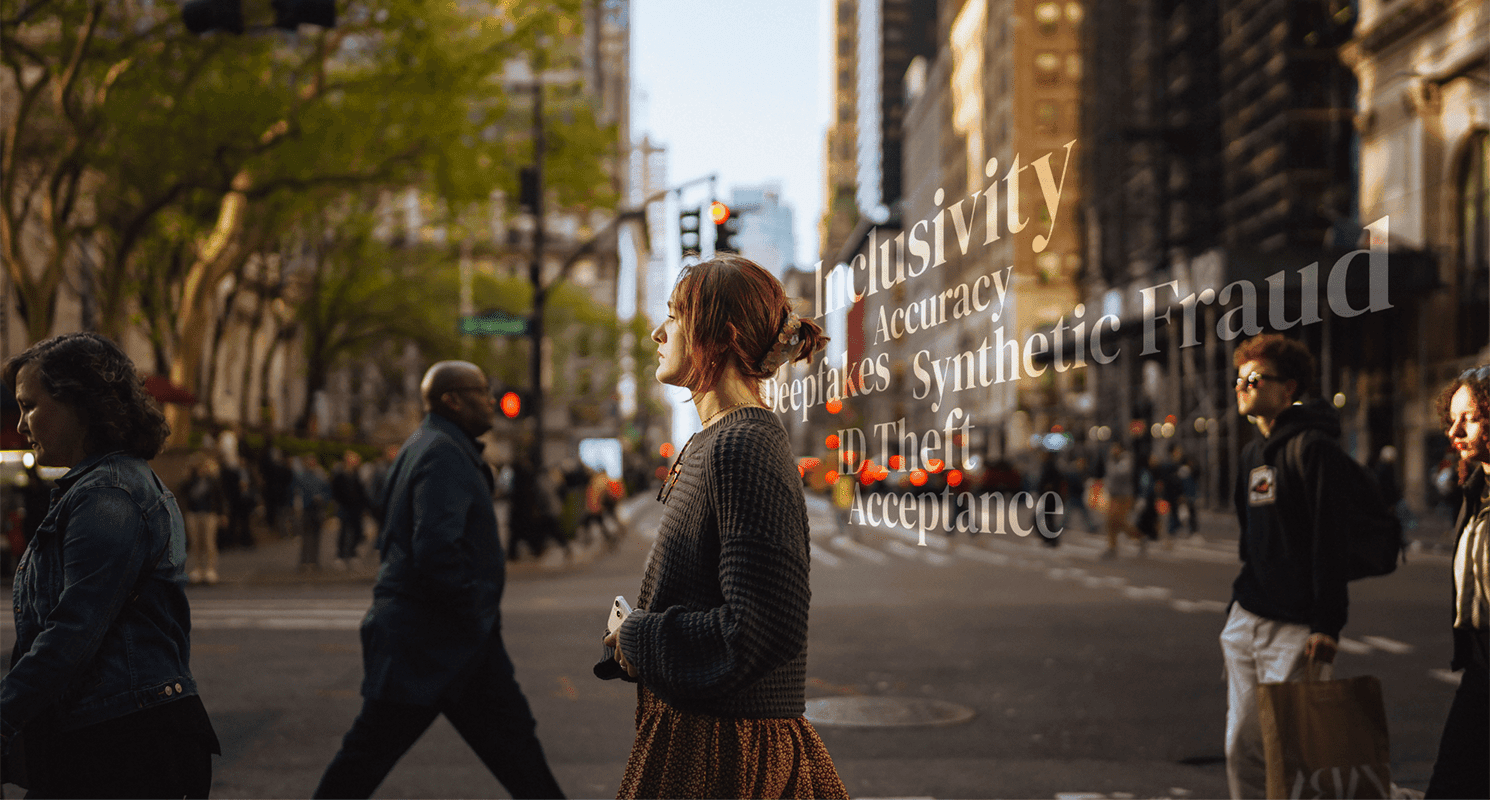
Let us prove it.
Scale your organization with confidence.